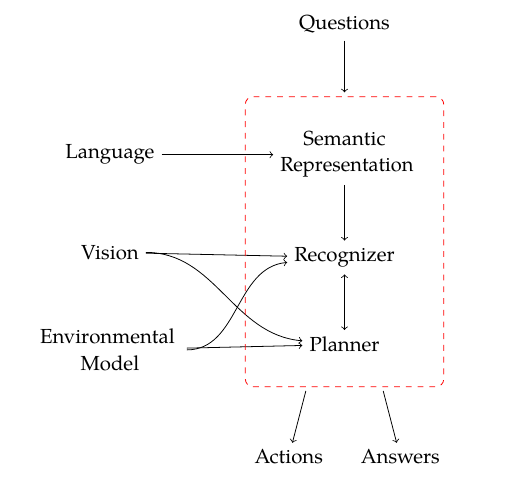
Safe and controllable autonomous robots must understand and reason about the behavior of the people in the environment and be able to interact with them. For example, an autonomous car must understand the intent of other drivers and the subtle cues that signal their mental state. While much attention has been paid to basic enabling technologies like navigation, sensing, and motion planning, the social interaction between robots and humans has been largely overlooked. As robots grow more capable, this problem grows in magnitude since they become harder to manually control and understand.
We are addressing this problem by combining language understanding, perception, and planning into a single coherent approach. Language provides the interface that humans use to request actions, explain why an action was taken, inquire about the future, and more. By combining this with perception one can focus the attention of a machine on a particular part of the environment, use the environment to disambiguate language and to acquire new linguistic concepts, and much more. Planning provides the final piece of the puzzle. It not only allows machines to follow commands, but it also allows them to reason about the intent of other agents in the environment by assuming that they too are running similar but inaccessible planners. These planners are only observed indirectly through the actions and statements of those agents. In this way a joint language, vision, and planning approach enables machines to understand the physical and social world around them and to communicate that understanding in natural language.
In the first phase of this project, we addressed the problem enabling a robot to jointly reason about what actions it should take in future with what it knows about the workspace from visual observations as well as language utterances from a human. Present language understanding models do not have the ability to acquire knowledge about past events or understand facts from a human that may be relevant later. We introduced a novel probabilistic model that allows us to reason with past context and acquire knowledge over time. This is accomplished by presenting an incremental approach that combines event recognition, semantic parsing, state keeping and reasoning about future actions of the robot. We demonstrate the model on a Baxter Research Robot.
Our ongoing work focuses on enhancing the model with the ability to perform deductive reasoning with the goal of enabling interpretation of instructions that necessitate logical inference over both rules and facts acquired by the robot. Our model also allows the robot to answer queries based on knowledge acquired from the workspace. Further, the robot can query the human in case of multiple grounding hypothesis for clarification and uses the response to disambiguate an action. We are also exploring the ability to acquire new previously-unknown concepts related to object types be jointly learning a model of attributes described in language and visual attributes derived from detections. We are also working on integrating our model with Toyota's Human Support Robot in a simulation environment.
This is a continuation of the project "Using Vision and Language to Read Minds" by the same PIs.
Publications:
- Y.-L. Kuo, B. Katz, and A. Barbu, “Encoding formulas as deep networks: Reinforcement learning for zero-shot execution of LTL formulas,” in IROS 2020 (accepted).
- Y.-L. Kuo, B. Katz, and A. Barbu, “Deep compositional robotic planners that follow natural language commands,” in 2020 IEEE International Conference on Robotics and Automation (ICRA), 2020, doi: 10.1109/ICRA40945.2020.9197464 [Online]. Available: https://doi.org/10.1109/ICRA40945.2020.9197464
- A. Barbu, D. Mayo, J. Alverio, W. Luo, C. Wang, D. Gutfreund, J. Tenenbaum, and B. Katz, “ObjectNet: A large-scale bias-controlled dataset for pushing the limits of object recognition models,” in NeurIPS 2019, 2019 [Online]. Available: https://papers.nips.cc/paper/9142-objectnet-a-large-scale-bias-controlled-dataset-for-pushing-the-limits-of-object-recognition-models
- S. Roy, M. Noseworthy, R. Paul, D. Park, and N. Roy, “Leveraging Past References for Robust Language Grounding,” in Proceedings of the 23rd Conference on Computational Natural Language Learning (CoNLL), Hong Kong, China, 2019, pp. 430–440, doi: 10.18653/v1/K19-1040 [Online]. Available: https://doi.org/10.18653/v1/K19-1040
- D. Park, M. Noseworthy, R. Paul, S. Roy, and N. Roy, “Inferring Task Goals and Constraints using Bayesian Nonparametric Inverse Reinforcement Learning,” in CorL 2019, 2019, vol. 100, pp. 1005–1014 [Online]. Available: http://proceedings.mlr.press/v100/park20a.html
- M. Noseworthy, R. Paul, S. Roy, D. Park, and N. Roy, “Task-Conditioned Variational Autoencoders for Learning Movement Primitives,” in CoRL 2019, 2019, vol. 100, pp. 933–944 [Online]. Available: http://proceedings.mlr.press/v100/noseworthy20a.html
- B. Myanganbayar, C. Mata, G. Dekel, B. Katz, G. Ben-Yosef, and A. Barbu, “Partially Occluded Hands: A challenging new dataset for single-image hand pose estimation,” in 2018 Asian Conference for Computer Vision (ACCV 2018), 2018, p. 14 [Online]. Available: http://accv2018.net/program/
- C. Ross, A. Barbu, Y. Berzak, B. Myanganbayar, and B. Katz, “Grounding language acquisition by training semantic parsers using captioned videos,” in Proceedings of the 2018 Conference on Empirical Methods in Natural Language Processing, 2018 [Online]. Available: http://aclweb.org/anthology/D18-1285
- Y.-L. Kuo, A. Barbu, and B. Katz, “Deep sequential models for sampling-based planning,” in IROS 2018, 2018 [Online]. Available: https://doi.org/10.1109/IROS.2018.8593947
- R. Paul, A. Barbu, S. Felshin, B. Katz, and N. Roy, “Temporal Grounding Graphs for Language Understanding with Accrued Visual-Linguistic Context (Cross-Submission with IJCAI 2017),” in The 1st Workshop on Language Grounding for Robotics at ACL 2017, Vancouver, Canada, 2017 [Online]. Available: https://robonlp2017.github.io/schedule.html
- R. Paul, A. Barbu, S. Felshin, B. Katz, and N. Roy, “Temporal Grounding Graphs for Language Understanding with Accrued Visual-Linguistic Context,” in International Joint Conference on Artificial Intelligence (IJCAI), Melbourne, Australia, 2017 [Online]. Available: https://doi.org/10.24963/ijcai.2017/629
- J. Arkin, R. Paul, D. Park, S. Roy, N. Roy, and T. M. Howard, “Real-Time Human-Robot Communication for Manipulation Tasks in Partially Observed Environments,” in 2018 International Symposium on Experimental Robotics (ISER 2018), Buenos Aires, 2018, p. 6 [Online]. Available: http://iser2018.org/
- D. Nyga, S. Roy, R. Paul, D. Park, M. Pomarlan, M. Beetz, and N. Roy, “Grounding Robot Plans from Natural Language Instructions with Incomplete World Knowledge,” in 2nd Conference on Robot Learning (CoRL 2018), Zurich, Switzerland., 2018 [Online]. Available: http://proceedings.mlr.press/v87/nyga18a.html
- M. Tucker, A. Derya, R. Paul, G. Stein, and N. Roy, “Learning Unknown Groundings for Natural Language Interaction with Mobile Robots,” in International Symposium on Robotics Research 2017, Puerto Varas, Chile, 2017 [Online]. Available: https://parasol.tamu.edu/isrr/isrr2017/program.php
- N. Glabinski, R. Paul, and N. Roy, “Grounding Natural Language Instructions with Unknown Object References using Learned Visual Attributes,” in AAAI Fall Symposium on Natural Communication for Human-Robot Collaboration (NCHRC), 2017, 2017.