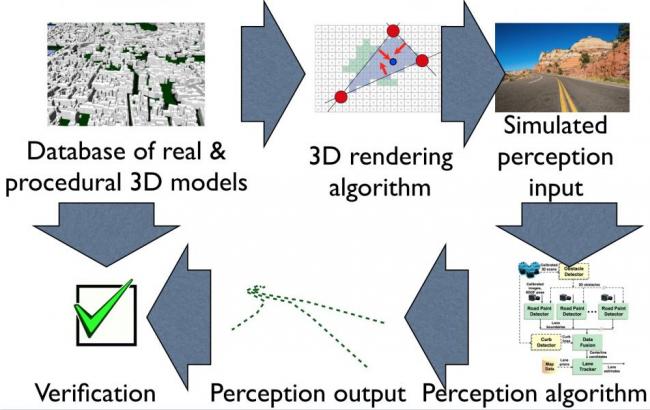
We aim to rigorously characterize the sensitivity of vision-in-the-loop driving controllers in increasingly complex visual tasks. While rooftop lidar provides a spectacular amount of high-rate geometric data about environment, there are a number of tasks in an autonomous driving system where camera-based vision will inevitably play a dominant role: dealing with lane markings and road signs, dealing with water/snow and other inclement weather conditions that can confuse a lidar, and even dealing with construction (orange cones), police officers, and pedestrians/animals. Furthermore, vision sensors are often fused with depth returns from a laser and other sensors as a part of the vehicle and obstacle estimation algorithms.
As an initial study, we will investigate the performance of a simple perception algorithm for lane detection and a simple controller for lane following, given visual scenes which capture some of the diversity of urban driving conditions here in Boston including complex on-road traffic markings at intersections and worn visual features. As the project progresses, we will attempt to simulate more and more of the visual world -- up to and including difficult volumetric effects such as fog or snow and dynamic obstacles such as pedestrians and other vehicles.
This is a continuation of the project "Simulation and Verification for Vision-in-the-Loop Control" by Fredo Durand.
Publications:
- S. P. Bangaru, T.-M. Li, and F. Durand, “Unbiased Warped-Area Sampling for Differentiable Rendering,” ACM Trans. Graph, vol. 39, no. 6, p. 18, Dec. 2020, doi: 10.1145/3414685.3417833. [Online]. Available: https://doi.org/10.1145/3414685.3417833
- Y. Hu, L. Anderson, T.-M. Li, Q. Sun, N. Carr, J. Ragan-Kelley, and F. Durand, “DIFFTAICHI: DIFFERENTIABLE PROGRAMMING FOR PHYSICAL SIMULATION,” in ICLR 2020, 2020 [Online]. Available: https://iclr.cc/virtual_2020/poster_B1eB5xSFvr.html
- T.-M. Li, M. Aittala, F. Durand, and J. Lehtinen, “Differentiable Monte Carlo Ray Tracing through Edge Sampling,” in ACM Trans. Graph. (Proc. SIGGRAPH Asia), 2018, vol. 37, pp. 222:1–222:11 [Online]. Available: https://doi.org/10.1145/3272127.3275109
- T.-M. Li, M. Gharbi, A. Adams, F. Durand, and J. Ragan-Kelley, “Differentiable programming for image processing and deep learning in halide,” ACM Trans. Graph., vol. 37, no. 4, pp. 1–13, Jul. 2018 [Online]. Available: https://doi.org/10.1145/3197517.3201383. [Accessed: 16-Sep-2019]
- M. Gharbi, J. Chen, J. Barron, S. Hasinoff, and F. Durand, “Deep Bilateral Learning for Real-Time Image Enhancement,” ACM Transactions on Graphics, vol. 36, no. 4, Jul. 2017 [Online]. Available: https://doi.org/10.1145/3072959.3073592, https://groups.csail.mit.edu/graphics/hdrnet/
- M. Gharbi, T.-M. Li, M. Aittala, J. Lehtinen, and F. Durand, “Sample-based Monte Carlo denoising using a kernel-splatting network,” ACM Transactions on Graphics, vol. 38, no. 4, pp. 1–12, Jul. 2019 [Online]. Available: https://doi.org/10.1145/3306346.3322954
- L. Anderson, T.-M. Li, J. Lehtinen, and F. Durand, “Aether: An Embedded Domain Specific Sampling Language for Monte Carlo Rendering,” ACM Transactions on Graphics (TOG), vol. 36, no. 4, Jul. 2017, doi: 10.1145/3072959.3073704. [Online]. Available: https://doi.org/10.1145/3072959.3073704
- A. Zlateski, R. Jaroensri, P. Sharma, and F. Durand, “On the Importance of Label Quality for Semantic Segmentation,” in IEEE CVPR 2018, 2018 [Online]. Available: https://doi.org/10.1109/CVPR.2018.00160
Videos: