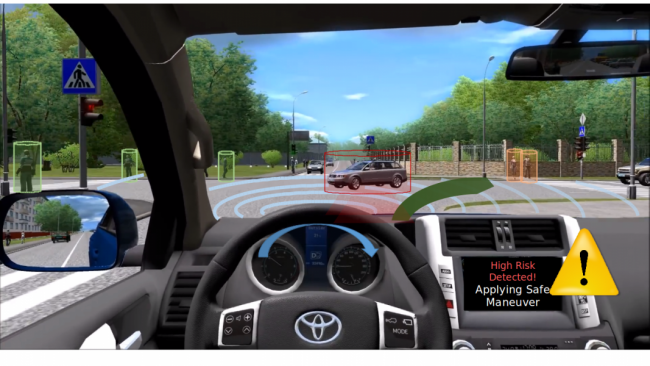
Despite improvements in automobile technology and comfort, modern driving is an unnecessarily stressful and dangerous activity. The primary reason for this is cognitive overload on the driver at multiple levels. The Toyota-CSAIL partnership puts forward the exciting vision of safer and less stressful driving through a “parallel” autonomous system that assists the driver by watching for risky situations, and by helping the driver take proactive, compensating actions before they become crises. The parallel autonomous system should also be able to jump in to save the driver in the event of an imminent accident. Fundamental to this endeavor is the ability to assess and manage risks. This includes 1) risks that pedestrians and other vehicles present to the driver, 2) the resulting risks of maneuvers that the driver’s vehicle selects in response, and 3) risks incurred by the coupling between the vehicle’s maneuvers and the likely responses by other vehicles and pedestrians.
A useful metaphor for this problem is a dance, where a leader (the driver) and a partner (the parallel autonomous system) collaborate to negotiate the dance floor environment, where numerous other couples are moving about. The leader sets the general plan, but a skilled partner will assert control when needed to avoid collisions with couples the leader cannot see. In a driving scenario, the “partner” parallel autonomous system should monitor the environment for pedestrians, other vehicles, and fixed obstacles, and predict the behavior of the external entities. It should also monitor the driver, predict the driver’s likely response to potential environmental hazards, and suggest or take adaptive actions. To do this the autonomous system must be aware of risk at all levels.
Consider the situation of vehicles entering a highway via an on-ramp. Studies show that highway ramp interchanges are the sites of far more accidents per mile driven than other segments of highways. Suppose, for example, that our vehicle is on a two-lane highway, approaching an entrance ramp, and another vehicle is entering the highway at the same time. The autonomous system must be aware of this situation, and must be able to predict likely possible outcomes. It must then be able to suggest or take adaptive actions. For example, our vehicle should avoid potential collision by changing lanes, accelerating to move ahead of the other vehicle, or decelerating to let the other vehicle proceed ahead. The parallel autonomous system would be aware of situations that limit these options, and thereby increase risk. For example, if there is a third vehicle in the left lane, parallel with our vehicle, the option of changing lanes may become difficult. In this case, the autonomous system might proactively decelerate the vehicle to reduce the risk, or nudge the driver through visual or haptic feedback.
In addition to risk-aware monitoring, predicting, and adapting, the parallel autonomous system must take actions collaboratively with the driver. In particular, transitions of control authority must be smooth. Just as in dancing, where the follower may temporarily assert control to guide the couple away from a collision, the parallel autonomous system must assert and relinquish control in a manner that does not upset the driver.
Publications:
- A. M. Jasour, A. Hofmann, and B. C. Williams, “Moment-Sum-Of-Squares Approach for Fast Risk Estimation in Uncertain Environments,” in IEEE CDC 2018, 2018 [Online]. Available: https://doi.org/10.1109/CDC.2018.8618744
- X. Huang, A. Jasour, M. Deyo, A. Hofmann, and B. C. Williams, “Hybrid Risk-Aware Conditional Planning with Applications in Autonomous Vehicles,” in IEEE CDC 2018, 2018 [Online]. Available: https://doi.org/10.1109/CDC.2018.8619771
- X. Huang, S. Hong, A. Hofmann, and B. C. Williams, “Online Risk-Bounded Motion Planning for Autonomous Vehicles in Dynamic Environments,” in Proceedings of the Twenty-Ninth International Conference on Automated Planning and Scheduling (ICAPS 2019), 2019 [Online]. Available: https://aaai.org/ojs/index.php/ICAPS/article/view/3479
- X. Huang, S. G. McGill, B. C. Williams, L. Fletcher, and G. Rosman, “Uncertainty-Aware Driver Trajectory Prediction at Urban Intersections,” in 2019 International Conference on Robotics and Automation (ICRA), Montreal, QC, Canada, 2019, pp. 9718–9724 [Online]. Available: https://doi.org/10.1109/ICRA.2019.8794282. [Accessed: 16-Sep-2019]