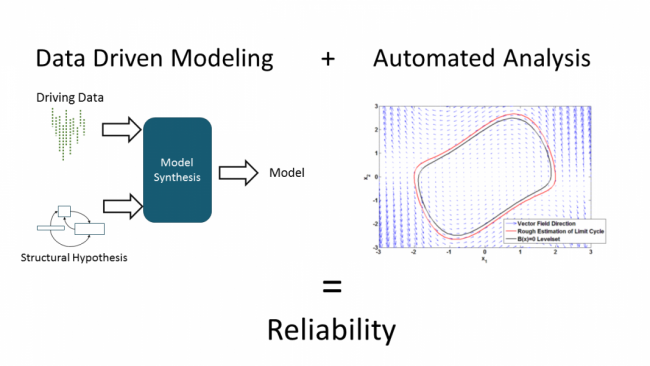
Autonomous cars face two major challenges. First, the system needs to analyze large amounts of data from its dynamic environment, and react intelligently and quickly. Second, any such reaction needs to be safe; even a small probability of accident per mile can be catastrophic in a mass deployment. To achieve these two goals, our project aims to develop a unified framework that brings together the advances in machine learning and control that can make autonomous driving possible with the advances in verification that can make it safe. Our goal is to be able to rigorously characterize the safety envelopes of a real-world autonomous driving solution by combining data driven modeling of complex urban environments with new capabilities for analyzing complex control systems.
This is a continuation of the project "Formal Verification Meets Big Data Intelligence in the Trillion Miles Challenge" by Armando Solar-Lezama, Russ Tedrake.
Publications:
-
J. P. Inala, A. Solar-Lezama, O. Bastani, and Z. Tavares, “SYNTHESIZING PROGRAMMATIC POLICIES THAT INDUCTIVELY GENERALIZE,” in ICLR 2020, 2020, p. 21 [Online]. Available: https://iclr.cc/virtual_2020/poster_S1l8oANFDH.html
-
S. Gao, J. Kapinski, J. Deshmukh, N. Roohi, A. Solar-Lezama, N. Arechiga, and S. Kong, “Numerically-Robust Inductive Proof Rules for Continuous Dynamical Systems,” in Computer Aided Verification, vol. 11562, I. Dillig and S. Tasiran, Eds. Cham: Springer International Publishing, 2019, pp. 137–154 [Online]. Available: https://doi.org/10.1007/978-3-030-25543-5_9.
-
O. Bastani, Y. Pu, and A. Solar-Lezama, “Verifiable Reinforcement Learning via Policy Extraction,” in NeurIPS 2018, 2018 [Online]. Available: https://papers.nips.cc/paper/7516-verifiable-reinforcement-learning-via-policy-extraction.pdf
-
S. Kong, A. Solar-Lezama, and S. Gao, “Delta-Decision Procedures for Exists-Forall Problems over the Reals,” in Computer Aided Verification, Cham, 2018, vol. 10982, pp. 219–235 [Online]. Available: https://doi.org/10.1007/978-3-319-96142-2_15