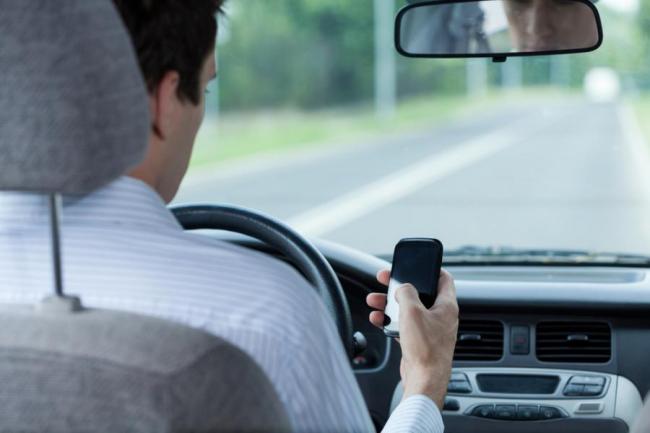
When is driving most risky? When do drivers find driving difficult or stressful? Why are these situations more risky or difficult for the driver? Should the car momentarily take over whenever the driver is distracted? Drivers are often distracted: they adjust the radio, talk to passengers, think about their day, and look at the scenery. If a semi-autonomous car were to take over whenever the driver were distracted, this might effectively require a fully autonomous vehicle! And having the vehicle assist during every distraction might be quite unnecessary, as clearly we often perform a wide range of driving tasks even while distracted. What driving tasks are most at risk, from what distracted behaviors, and why?
In developing an automated vehicle system to augment and complement the human driver, it is critical to understand what driving tasks are hardest for humans and would benefit the most from automation. We will learn the “pain points” in driving through a mix of computer vision, machine learning, and understanding of human vision and attention.
This is a continuation of the project "Uncovering the Pain Points in Driving" by Ruth Rosenholtz, Fredo Durand, William Freeman, Aude Oliva, Antonio Torralba.
Publications:
- B. Wolfe, B. D. Sawyer, and R. Rosenholtz, “Toward a Theory of Visual Information Acquisition in Driving,” Hum Factors, p. 001872082093969, Jul. 2020, doi: 10.1177/0018720820939693. [Online]. Available: https://doi.org/10.1177/0018720820939693
- B. Wolfe, B. Seppelt, B. Mehler, B. Reimer, and R. Rosenholtz, “Rapid holistic perception and evasion of road hazards.,” Journal of Experimental Psychology: General, Jul. 2019 [Online]. Available: https://doi.org/10.1037/xge0000665
- B. Wolfe, B. D. Sawyer, A. Kosovicheva, B. Reimer, and R. Rosenholtz, “Detection of brake lights while distracted: Separating peripheral vision from cognitive load,” Attention, Perception, & Psychophysics, Jun. 2019 [Online]. Available: https://doi.org/10.3758/s13414-019-01795-4
- B. Wolfe, L. Fridman, A. Kosovicheva, B. Seppelt, B. Mehler, B. Reimer, and R. Rosenholtz, “Predicting road scenes from brief views of driving video,” Journal of Vision, vol. 19, no. 5, p. 8, May 2019 [Online]. Available: https://doi.org/10.1167/19.5.8
- B. Wolfe, J. Dobres, R. Rosenholtz, and B. Reimer, “More than the Useful Field: Considering peripheral vision in driving,” Applied Ergonomics, vol. 65, pp. 316–325, Nov. 2017 [Online]. Available: https://doi.org/10.1016/j.apergo.2017.07.009
- B. Wolfe, L. Fridman, A. Kosovicheva, B. Seppelt, B. Mehler, R. Rosenholtz, and B. Reimer, “Perceiving the Roadway in the Blink of an Eye–Rapind Perception of the Road Environment and Prediction of Events,” in 9th International Driving Symposium on Human Factors in Driver Assessment, Training and Vehicle Design, Manchester Village, Vermont, 2017 [Online]. Available: http://drivingassessment.uiowa.edu/sites/default/files/DA2017/papers/33.pdf
- M. Monfort, A. Andonian, B. Zhou, K. Ramakrishnan, S. A. Bargal, T. Yan, L. Brown, Q. Fan, D. Gutfruend, C. Vondrick, and A. Oliva, “Moments in Time Dataset: one million videos for event understanding,” IEEE Transactions on Pattern Analysis and Machine Intelligence (PAMI), Feb. 2019 [Online]. Available: https://doi.org/10.1109/TPAMI.2019.2901464
- Z. Bylinskii, T. Judd, A. Oliva, A. Torralba, and F. Durand, “What do different evaluation metrics tell us about saliency models?,” IEEE Transactions on Pattern Analysis and Machine Intelligence, Mar. 2018 [Online]. Available: https://doi.org/10.1109/TPAMI.2018.2815601
- N. W. Kim, Z. Bylinskii, M. A. Borkin, K. Z. Gajos, A. Oliva, F. Durand, and H. Pfister, “BubbleView: a validation of a mouse-contingent interface for crowdsourcing image importance and tracking visual attention,” ACM Transactions on Computer-Human Interaction (TOCHI), vol. 24, no. 5, pp. 36:1-36:40, Nov. 2017 [Online]. Available: https://doi.org/10.1145/3131275
- M. Monfort, M. Johnson, A. Oliva, and K. Hofmann, “Asynchronous Data Aggregation for Training End to End Visual Control Networks,” in Proceedings of the 16th Conference on Autonomous Agents and MultiAgent Systems, São Paulo, Brazil, 2017, pp. 530–537 [Online]. Available: http://dl.acm.org/citation.cfm?id=3091125.3091204